If you haven’t rewatched the epic finishing-straight duel between Paul Tergat and Haile Gebrselassie from the 2000 Olympics recently, do yourself a favor and click here. Okay, now you’re in the mood for one of the perennial running debates: where do amazing kickers like Geb get their amazing kicks from?
There are three main schools of thought. One is that sprint speed is the prerequisite for a fast sprint finish, an idea recently fleshed out by proponents of the speed reserve concept. A second is that the fastest finishers are simply those who are least tired at the end of the race, so great endurance is the key. A third is that it’s all in your head—that Geb’s ability to narrowly outlean Tergat nearly every time they raced is best explained by differences in self-belief rather than physiology.
But maybe there’s a better explanation. A team of researchers led by Brett Kirby of Nike Sport Research Lab, along with collaborators including Andrew Jones of the University of Exeter, who worked with Kirby on Nike’s Breaking2 project, recently published a paper in the Journal of Applied Physiology that uses a simple mathematical model to predict how pacing strategies affect runners with different strengths and weaknesses. The model produces a whole pile of interesting insights, but the one that grabbed my attention was its ability to accurately predict how fast each runner will run the final lap of a given race.
The paper was inspired by the men’s 5,000- and 10,000-meter events at the 2017 World Championships, where the overwhelming favorite Mo Farah, unbeaten at major championships over those distances since 2011, defended his 10,000 title but was outkicked by Muktar Edris in the 5,000. Was there something in the way the two races played out that produced those results? And more importantly, the researchers wondered, could the outcomes have been predicted in advance?
The model that Kirby and his colleagues use relies on a concept called critical speed. I’ve written about it a few times before, and the full study is free to read online for those who want to dig into the details. For our purposes, critical speed is basically a threshold that divides metabolically sustainable efforts from unsustainable ones. Once you’re going faster than critical speed, as races between 800 and 10,000 meters inevitably do, the clock is ticking down to your eventual exhaustion. How long that takes, or equivalently how much energy you can expend above that critical threshold, depends on a second parameter—a sort of spare fuel tank—that is sometimes called anaerobic capacity. (The terminology is controversial for various technical reasons, but I’m going stick with anaerobic capacity because I don’t know of any better options. In the paper, they just call it D’, and it’s expressed in units of distance. I like to think of it as the maximum distance you could sprint before keeling over if you held your breath, but that’s a metaphor rather than a physiological explanation.)
The paper analyzes the results of both the 5,000- and 10,000-meter races from those 2017 championships. For each athlete, the researchers calculate a critical speed and an anaerobic capacity based on previous race results (as described here). Those parameters give you a prediction of who would win the race—but that prediction assumes that everyone is going to run a perfectly even pace that maximizes their personal capabilities, by running just enough faster than their critical speed to exhaust their anaerobic capacity as they cross the finish line.
That’s not how things work in the real world, though—because the pace varies constantly depending on who’s leading and what tactics the runners are employing. If the initial pace is fast, it will force runners to start burning up their anaerobic reserve right away, which favors competitors with high critical speed. If the initial pace is slow, then the race will come down to a late burn-up that favors those with high anaerobic capacity. This isn’t a particularly deep insight: fast races favor aerobic monsters and slow races favor kickers.
But real-life championship races are seldom all fast or all slow; the pace varies constantly as runners surge, relax, and counterattack. Each runner’s unique anaerobic reserve is draining whenever the pace is faster than their unique critical speed, and recharging when the pace is slower. Using the lap-by-lap splits of the 2017 championship racers, Kirby and his colleagues are able to recalculate where each runner stands after every lap. At the start of the race, knowing the runners’ critical speed and anaerobic reserve doesn’t give you a very good prediction of what order they’ll eventually finish in. But with each passing lap, the prediction gets better and better—until, with 400 meters to go, the numbers give you a near-perfect forecast of how the race will play out.
In part, the prediction gets better because weaker runners drop off the pace as their anaerobic capacity hits zero. That’s what happened in the 10K, so there were only six men left in contention for the final lap. In the 5K, which was a slower, more tactical race, the entire field was still in the mix at the bell. In both cases, the finishing order—and, to a remarkable extent, the times for the final 400 meters—were predicted not by who was the fastest sprinter or had the best endurance, but by who had the most anaerobic capacity left at that exact moment in time, after 4,600 or 9,600 meters of surges and countersurges.
The result? With a lap to go in the 5K, Muktar Edris was favored to win, despite starting the race as the fourth seed, according to the model. Yomif Kejelcha, the model’s prerace favorite and the runner who, in real life, was leading the race as the final lap started, was now predicted to finish only fourth based on his depleted anaerobic capacity. Farah was picked for second, with American Paul Chelimo, who had fallen back to sixth, picked for third. That’s exactly how it played out: the model correctly predicted the places of all nine runners for whom it had sufficient pre-race data to estimate their critical speed and anaerobic capacity.
Here’s a graph showing time for the last lap as a function of anaerobic capacity remaining at the start of that lap (that’s D’, shown as a distance in meters):
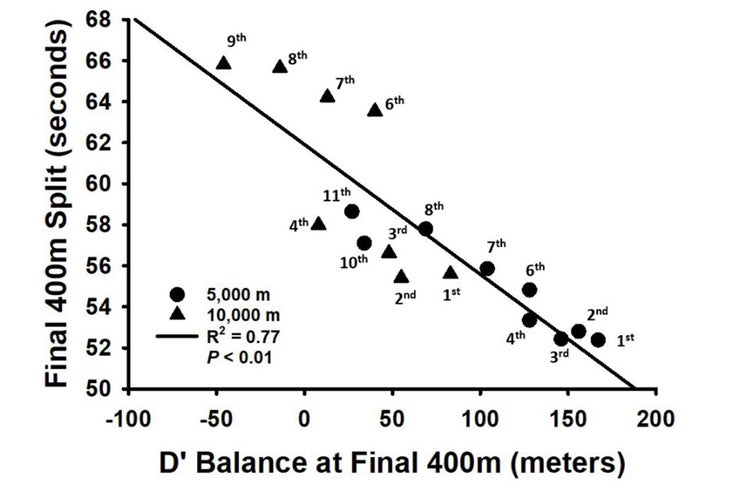
The greater the remaining anaerobic capacity, the faster the last lap. It’s not perfect: you can see that the third-place finisher in the 5,000, Chelimo, actually closed marginally faster than the two men ahead of him, despite having less D’ to burn. But overall, it’s uncannily accurate at predicting the notoriously hard-to-predict finishing kick.
The key point here is that neither endurance nor sprint speed, on their own, would have pegged Farah as a near-unbeatable championship runner for six years. He didn’t have the highest critical speed in either the 5K (that was Kejelcha) or the 10K (that was Kenya’s Paul Tanui). He had only the fourth-biggest anaerobic capacity in both the 5K and 10K. But he somehow mastered the art of riding the razor’s edge of his critical speed and controlling the closing stages of races in order to reach the last lap with the most anaerobic capacity left.
The point? Perhaps if you know someone’s critical speed and anaerobic capacity (which can be estimated from their best race times at three different distances), you can devise the best strategy to beat them, depending on whether you have a better critical speed or anaerobic capacity. Perhaps, for better or worse, we’ll eventually have real-time estimates of anaerobic capacity displayed on our wrists as we race. But I’ll confess: no matter how many times I watch Geb reel in and then outlean Tergat, I’m still not convinced there’s any physiological model that can fully capture that magic.
For more Sweat Science, join me on Twitter and Facebook, sign up for the email newsletter, and check out my book Endure: Mind, Body, and the Curiously Elastic Limits of Human Performance.